The Derivative Integral Proportional Controller (PID) was created in 1911 by inventor Elmer Sperry, initially for the United States Navy, with the aim of automating the steering of ships and imitating the behavior of a helmsman, capable of compensating and predicting constant variations on the high seas. Years later, engineering published the first theoretical analysis of this control, describing it through a mathematical equation that is the basis of calculus today.
PID is one of the most widely used control techniques in the industry, ensuring the stability and performance of processes. Whether in temperature, pressure, flow or level regulation, the PID stands out for its versatility and efficiency. Each component constitutes a precise control of the system:
- Proportional Control (P): Generates an output signal proportional to the current error, which is the difference between the desired value (setpoint) and the measured value (process). This action helps reduce the error, but it does not eliminate it completely.
- Integral Control (I): Produces an output signal proportional to the magnitude and duration of the error, thus eliminating the accumulated error.
- Derivative Control (D): Considers the rate of change of the error, helping to predict its future behavior and apply faster corrections, improving system stability and reducing oscillation.
However, the configuration and monitoring of these controllers can be improved, especially through integration with innovative technologies such as data science. The lack of visibility into the performance of PIDs and the difficulty in identifying opportunities for improvement can lead to problems such as process instability, wasted energy, and increased maintenance costs.
PID Graphical Visualization: Precise and Efficient Adjustments
The PID controller can be graphed, exemplifying the system’s response over time. To do this, it is built as follows:
- X-axis: Represents time.
- Y-axis: Represents the value of the variable being controlled, such as temperature, pressure, speed, etc.
- Setpoint Line: A constant horizontal line that represents the desired value (setpoint) of the tracked variable.
- System Response Line: A curved line that shows how the controlled variable changes over time in response to PID control. It starts at the initial value of the controlled variable and moves until it eventually stabilizes at the setpoint value.
Importantly, if the response line exceeds the setpoint value, the process is called an “overshoot”. If it falls below the setpoint value, it is called an “undershoot”. The PID controller is used to minimize these variations.
The evaluation of the performance of the PID is done through some parameters:
- Rise Time: The time it takes for the system to reach the setpoint value for the first time.
- Settling Time: The time it takes for the system’s response to stabilize at the setpoint value.
- Steady State Error: The difference between the setpoint value and the final value of the system response.
The figure below illustrates a graphical example of the action of a PID controller:
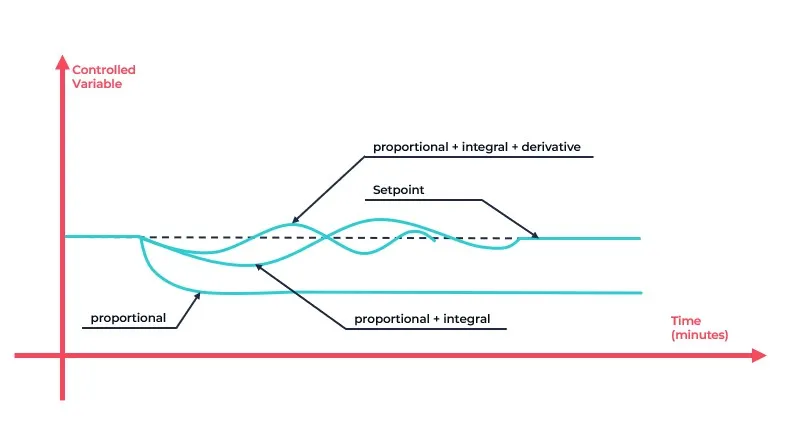
Industrial Automation with PID Controller
The PID controller is an essential tool in industrial automation. According to the Federal Institute of São Paulo, in the study “PID controller and its applications” (2021), about 97% of industrial applications use this technology. PID controllers are usually present in Logic-Programmable Controllers (PLCs) and are designed for specific control tasks that require continuous modulation.
In industry, the PID controller can be used to control several variables, such as:
- Temperature control: In industrial ovens and machines in general, through the reading of temperature sensors.
- Liquid level monitoring: In storage tanks, adjusting the flow rate of
- Pressure control: In hydraulic systems, adjusting the pump or relief valve within the desired range.
- Speed adjustment: In machines, setting the delivery of inputs within the ideal parameter.
However, extracting information from a PID controller in a PLC presents some challenges. It is necessary to establish a harmony between the parameters, that is, to find the ideal values for the proportional, integral and derivative constants. This requires in-depth technical knowledge of the systems and careful calibration. It is also necessary to clearly identify which variables are controlled and manipulated, and detect errors when there is a difference between them and the desired value.
To enhance and take advantage of the functionalities of a PID controller, the industry must collect and analyze the data generated. This data, coming from sensors and connected devices, helps to monitor system performance, identify bottlenecks, and optimize the production process.
PID Optimization with Data Analytics
Utilizing statistical techniques to collect and analyze data from PID controllers in real time is a powerful strategy for optimizing industrial performance. This analysis allows you to identify patterns, trends, and anomalies that can affect control, enabling precise and efficient adjustments to PID parameters. This ensures more stable, precise and cost-effective control.
To evaluate the performance of PIDs, some metrics are essential. The maintenance score, for example, indicates the need for preventive or corrective maintenance of the controller or actuator (such as pumps and inverters), based on indicators of control saturation. The fit score, on the other hand, evaluates the quality of the PID adjustment, considering stability, speed of response, and steady-state error.
Clear, customizable visualization of data is critical for intuitive analysis. Customizable dashboards allow operators and engineers to monitor PID performance in real time, quickly identify problems, and make informed decisions. With data science, it is possible to get an overview of the performance of PIDs, keep track of all plant controllers in one place, and identify problems through automatic alerts and notifications about setpoint deviations, control instability, or maintenance needs.
In addition, benchmarking makes it possible to compare the performance of different controllers, from best to worst, and implement improvements. Historical data allows you to track the evolution of PIDs’ performance over time and assess the impact of optimization actions.
Integrating data science with PID control not only improves the efficiency and stability of industrial processes, but also promotes smarter and more proactive management, raising the productivity and sustainability of the industry.
PID and Real-Time Data for an Optimized Industry
An industry that uses the PID controller stands out positively compared to one that does not. With PID, it is possible to have a more precise control of the error between the desired value and the actual value of a system variable. In addition, a production line equipped with this type of controller responds more effectively to changes in production conditions, adjusting quickly to keep the controlled variable close to the desired value.
As a consequence, the industry as a whole experiences an improvement in operational efficiency, especially when there is data collection and analysis from a system with a PID controller. This allows you to evaluate all controller parameters (proportional, integral, and derivative) and their performance over time. Access to this information provides an overview of the operation, serving as a basis for more assertive improvements.
The integration of data science with PID control represents a true revolution. With the union of these two tools, it is possible to obtain greater harmony between the parameters, an adaptive control and simulations of the future behavior of the system. By providing accurate, real-time information on the performance of controllers, the data enables industries to optimize their processes, reduce costs, and increase efficiency.
Learn more about ST-One.