Discover which data is relevant: creating data within the industry
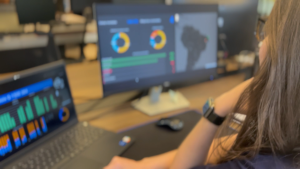
Photo: ST-One – rights reserved
Within the industry, day to day life is an intense demand for better results, and this takes place in several ways: To achieve the desired production goals, increased machine performance, better use of inputs and the more sustainable use of natural resources .
To maintain a high-performance industry, which operates within a high standard of efficiency, it is essential to use data science to monitor the most important indicators. With it, it is possible to study and make decisions based on the factory floor – understanding its perspectives -, provide accurate information about the production line, monitor and have control over the uninterrupted quality status, understand water and energy consumption, among others. .
Despite the notable advantages of industry digitalization, according to the study Data Paradox (2021) – carried out by the consultancy Forrester, and commissioned by Dell Technologies –, in the world only 66% of companies use data, and only 21% of this total know how to make the most of them appropriately to generate results. In Brazil this scenario is not very different, as in 2016 – according to a study by the National Confederation of Industry (CNI), called Special Welding on Industry 4.0 in Brazil (2021) – only 48% of industries made use of some digital technology in a given production stage, in 2021 this percentage rose to 69%. Most of them are still in the initial process, known as “data newbies”, and still don’t know very well how to deal with such access to information.
In short, tracking relevant metrics intelligently is essential for increasing factory productivity. According to the article “ Understand why data analysis is essential for the industry”, published in 2021 by the website Voz da Indústria, “structuring good data analysis guarantees the industry the possibility of making much better decisions assertive and based on reliable information, which represents the true scenario”, which reinforces the importance of such KPIs.
How an industry handles data
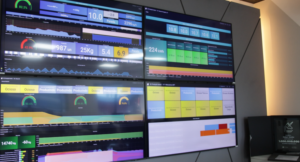
Foto ST-One – direitos reservados
The data is not always available and formatted in an easy-to-interpret model that contributes to assertive decision-making. Reports are common that the volume of information collected within the industry is growing, but this is not categorized, hierarchized and much less integrated – as illustrated in the article “Data utilization in the manufacturing sector – four challenges and solutions explained</ a>” published by the STRATUS Blog in 2023. From this frustration, many managers are faced with the question: “What data should I look at?”
To answer this question, it is necessary, right from the start, to define which analysis should be carried out, and check whether its objective is aligned with the strategic goals of the business. After that, it is necessary to understand what data is available to then get to the main point of the issue, which is identifying the relevant data.
However, several aspects of the routine of a production process complicate the clear visualization and understanding of important data. Some would be, having a manufacturing park with machines using different technologies, low-resolution manual processes (with incomplete information) or even time gaps between one piece of data and another, which prevents a stable analysis.
Often the most important data is not easily available, but that does not mean it cannot be found. In these situations, the data scientist (find out here how becoming one), through the right tools, you can create the data that makes the most sense to be monitored – based on the combination of two or more variables that are possible to be extracted natively from the factory floor.
Creating data from data
One way to create the data that needs to be monitored is to use formulas with the available information to calculate the key indicator. The following paragraphs detail a process in which this feature is used.
In an industry, improving the performance of a production line often means innovating: through different management practices, new technologies, efficient designs, rethinking the use of inputs, etc. According to the article “Productivity in industry</ a>” from Industry Portal (2022), “There are no limits to innovation, therefore, there are no limits to increased productivity”.
To carry out productivity analysis, it is necessary to measure and compare performance indicators, such as production quantity and speed, among other pre-defined standards. Thus, it is possible to identify areas for improvement, implement corrective actions and monitor the impact of these actions on the factory’s productivity and overall performance.
In this example, 2 machines will be considered that, for around 40 minutes, were producing at the same time (same availability), and which obtained the following results:
Machine 1: 10,287 products
Machine 2: 11,120 products
When comparing the results, there is a difference of almost 8%, which will impact the results for the entire month if not resolved. When searching for productivity data from these machines, the following information is obtained:
When analyzing the graphs separately, it is challenging to find where the problem is and how it is possible to optimize the use of the machine that has low performance. Therefore, one of the techniques to be applied is the combination of both information into a single image, which, although it does not leave a clear conclusion, allows the comparison of performance:
Data found: how to perform a relevant analysis
Within the productivity analysis, one of the indicators that can be monitored is the speed of the machines, as previously mentioned. Since production data is collected every 5 seconds, it is possible, for example, to calculate the speed every 30 seconds with the formula:
Speed=∆Production/∆Time
Even though the “speed” variable cannot have been natively extracted from the machinery, it is possible to discover it and look only at the most relevant data to understand the line’s performance. The answer to the question mentioned a few paragraphs above “which one should I look at” is often nebulous precisely because the information that is sought cannot be collected directly from the equipment, but it can be created. When comparing, it becomes clear in which period there was the greatest discrepancy, and what can be done to improve performance.
In this comparison it is clear that the speed of machine 1 is lower than that of machine 2, and at no point can machine 1 reach the same speed level as machine 2, remaining around 6% lower at peak performance moments.
With this type of information, the work to be carried out to improve the line’s overall performance must focus on increasing the production speed of machine 1, with a gain margin of more than 6%. Machine 1 still does not operate as fast as machine 2, which is more efficient. With this, and with the discovery that it is possible to increase the speed of the first machine, the result is greater performance in less time.
This work needs to consider the nominal speeds of subsequent machines and in this case a V-Graph can be a great help tool.
Data that unlocks results
The use of data in industry is an increasingly present reality in Brazil and around the world. With increasing demand, digitalization allows factories to achieve greater production optimization, meeting needs and requirements in less time and in a more automated way.
For this to be done, it is necessary to monitor the right data, which is not always available natively. This does not mean that it cannot be found, on the contrary, with a team of data scientists it is possible to find the most appropriate calculation to reach the variable that must be observed, such as by comparing two variables or using formulas.
Although the case used is a performance analysis, this line of reasoning (data comparison) also works to monitor other areas, such as use of raw materials, natural resources, maintenance, product quality, etc. This scenario comes to fruition thanks to the range of possibilities allowed by data science and the analysis capacity of scientists, who use their experience, creativity, and market vision to find the most relevant metrics for the industry. Using methods like these means that data stops being just data and becomes information that unlocks results.
Discover how data science contributes to different applications:
Performance
Raw Material
Natural Resources
Maintenance and Quality
Research and Development