In the last year, ST-One collaborated with UFMG (Federal University of Minas Gerais) and M. Dias Branco, an important Brazilian food industry, in innovative projects. The objective was to develop research focused on the creation of machine learning models to optimize the margarine production process, specifically in the oil deodorization section.
This section is crucial as it removes substances that impair the odor, taste, and stability of the final product. The process begins with heating the oil, allowing it to be filtered. Then, water vapor is injected into the heated oil to absorb the fatty acids present, followed by a cooling to prevent oxidation. Finally, the oil undergoes vacuum distillation, removing volatile components that cause instabilities in the product.
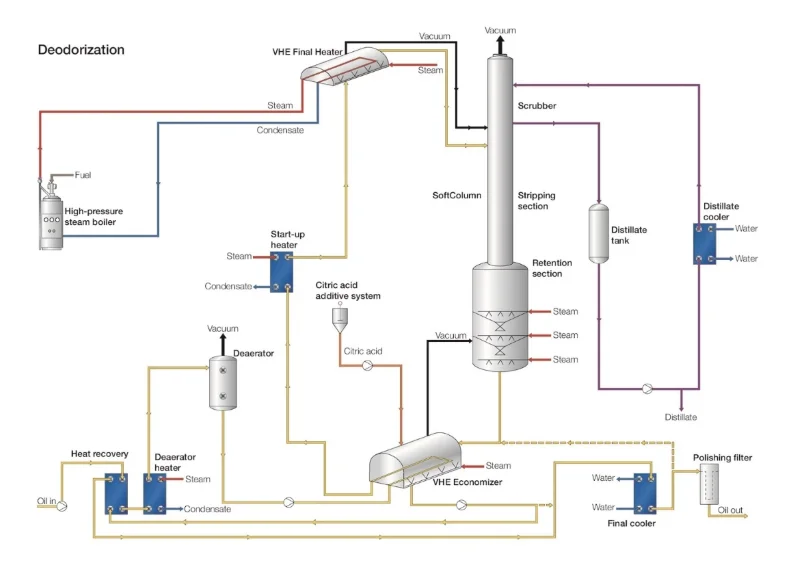
Vacuum is essential at this stage, as it allows deodorization to occur at lower temperatures, reducing the risk of thermal degradation of the oil and increasing the efficiency of the process. Ensuring that this occurs without errors is vital to ensure that the margarine produced is safe and pleasant to the taste.
Margarine is an indispensable food in the diet of many Brazilians. According to a survey by the Brazilian Association of Nutrology (Abran), in 2011, about 32.2% of Brazilians opted for the consumption of margarine for breakfast.
The structure of the Partnership
Since March 2023, the research has focused on understanding the vacuum break time, which occurs when it exceeds a certain operational limit. The results will be used as a basis for implementing improvements in the production line. The research involved three institutions – ST-One, UFMG and M. Dias Branco – each playing an essential role in the search for more technology and innovation.
M. Dias Branco, one of the largest food industries in Brazil, is always looking for digitalization to improve its production process. It has an industrial unit specialized in the production of special fats and margarines, which served as a reference point and expertise for the project.
UFMG (Federal University of Minas Gerais), through the computer science department, has the renowned Artificial Intelligence Laboratory (LIA). In this laboratory, projects with scholarships focused on the use of machine learning and data processing are encouraged. LIA is a reference in the area, even collaborating with international institutions, such as Stanford University, in the United States. These tools are applied in several areas, including industry.
ST-One acted as the main sponsor, providing all the data necessary for the execution of the study, based on the technology it develops. Weekly meetings throughout the process allowed for a rich exchange between ST-One’s R&D lab and the other parties involved.
The solutions developed aim to build predictive models that help the operations team to act on the system before the vacuum breaks. The realization of the project resulted in greater knowledge and mastery of machine learning for all parties involved. In addition, it was an opportunity to apply the models created in an industrial environment, allowing the improvement of results.
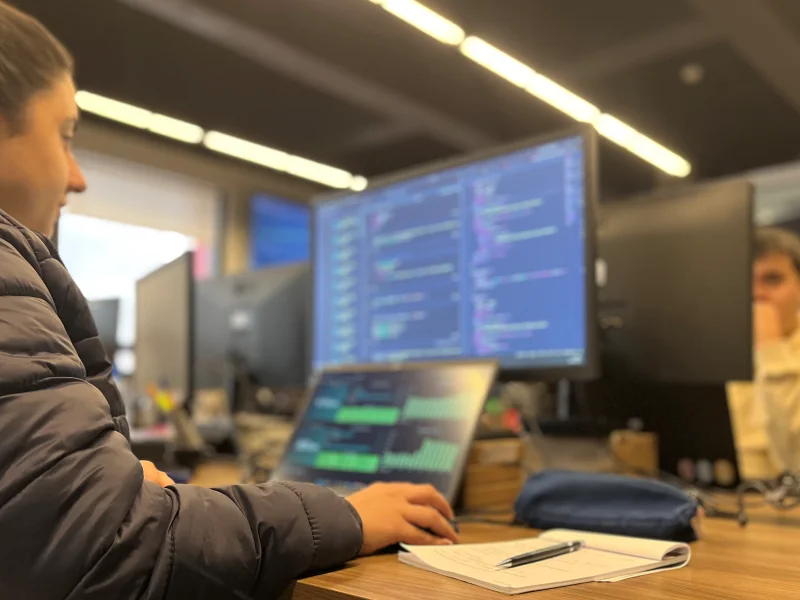
Vacuum Break Prediction: A Three-Phase Study
As mentioned, vacuum is a crucial part of the vegetable oil preparation process, used in the manufacture of margarine and fats in general. Throughout the project, three distinct machine learning models were created to test which one would provide the most accurate answers. This is important because creating a vacuum is a complex and costly process in case of an error.
With this in mind, the idea was to develop machine learning models capable of predicting the time until the vacuum breaks. The project was divided into three major stages: investigation, mathematical modeling, and testing of the results.
The first stage focuses on the analysis and identification of the issue to be worked on. This initial phase must be carefully planned, due to the model’s large contribution to production and the various nuances involved that determine success. The investigation involves defining the tools to be used, the models to be created and the ideal answers they should provide.
The second step involves applying different mathematical formulas to train these models, operationalizing the process and allowing its visualization. In this phase, a specific amount of data is separated to be submitted to the chosen machine learning model, which processes this data and transforms it into a trainable format.
LIA scientists developed questions to create a model that explains the behavior of the data collected. With this, the M. Dias Branco team was able to make adaptations in the automation of the equipment.
The last step of the project is the testing of the model, to ensure that, after training, the data patterns are recognized. This is done using a test dataset that the model has not yet seen. From the test results, new questions arise, especially if the process failed to identify the answers with the necessary accuracy. If all goes well, the model is implemented.
These steps result in a continuous cycle, using the “explanation” method developed by the LIA (Artificial Intelligence Laboratory) itself.
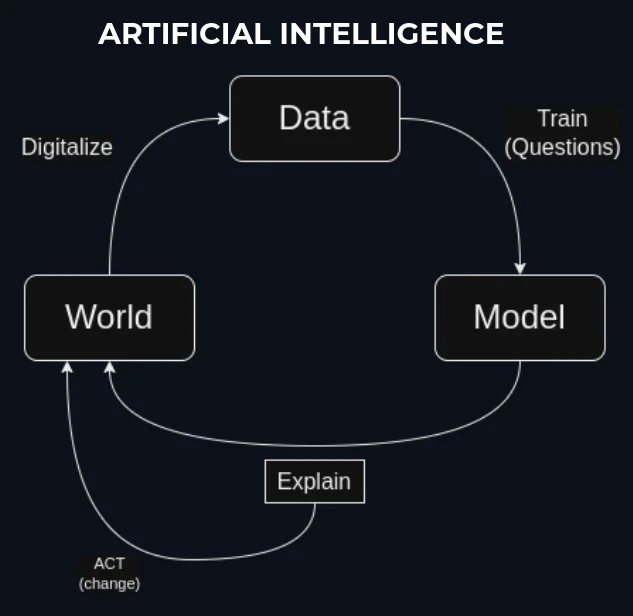
Academic and Industry Partnership: Machine Learning Outcomes
The models developed are still being applied, already bringing several beneficial results.
In the university environment, the data-academy initiative in conjunction with industries brings more innovation and opportunities. In the laboratories, the academics dedicate themselves to research aimed at development. These studies, with cutting-edge theoretical bases and innovations in the market, are applied in real cases in the industry, resulting in valuable practical experiences. In addition, the relationship with renowned educational institutions brings more resources to the industry.
For ST-One, in addition to the experience as a whole, the opportunity to delve into Artificial Intelligence domains was uplifting. By engaging in the exploration, model development, and application stages, it was possible to improve the interpretation of the “world” through the data and visualize it within its own framework. ST-One improves with each new challenge, always aiming to bring more technology and productivity.
The plant also gained more knowledge, contacts, and understood the possible ways to predict a vacuum break, given its complexity. This is a path to further development and retraining, until you reach the ideal amount of time. Thus, those responsible guarantee the quality of the process and a smarter and more assertive line.
Actions like this are productive for all parties, helping to keep students, professionals and those involved in the areas of data and industry always updated and seeking improvements. Learn more about ST-One.